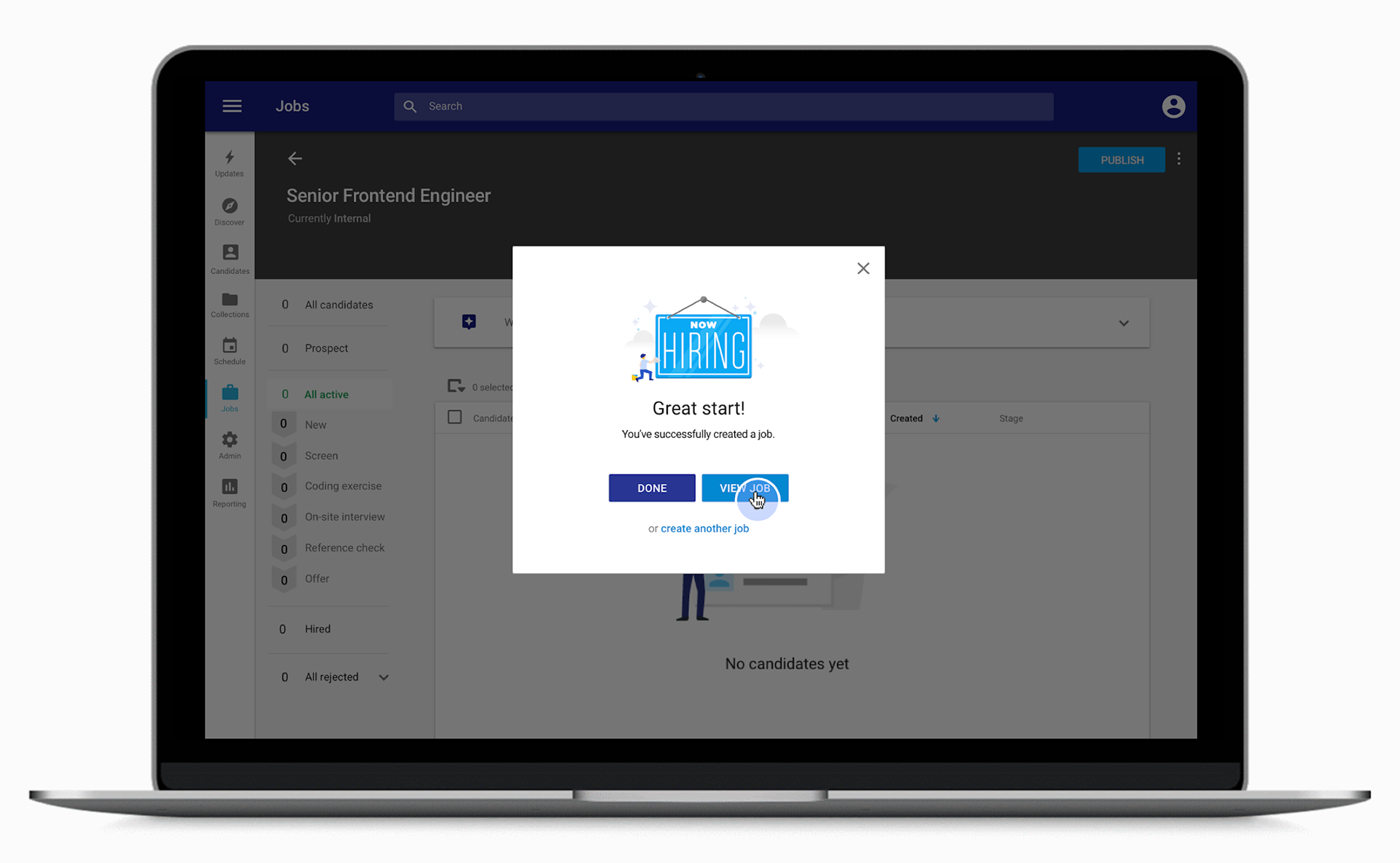
Problem
On average, companies engage with 250 candidates before finding the single candidate they want to hire. How might we capitalize on the 249 candidates who weren’t right for this role, but could be a good match for future openings?
User research
Our assumption as a pod was that past candidates would be useful to surface in new jobs. But in order to learn more about the potential value of these candidates, I wanted to talk with both recruiters and sourcers to learn more about how they approach these candidates today. We wanted to answer the following questions:
•Should recommendations include people who already applied and those who haven’t (in ATS)?
•How should the recommendation be presented?
•How many recommendations would you expect for a given job?
•What is the key optimization for these recommendations i.e. skillset, availability, likelihood to call back?
•When should recommendations be surfaced and where?
•What actions would you like to take on a given recommendation?
•In the case you don’t like a recommendation, what actions would you like to take?
•Should rationale for a recommendation be visible? If so, how?
Prior to the start of the research, I created some preliminary designs to show to recruiters and sourcers to gauge their initial impressions of a 'smart' recommendation feature. I experimented with a variety of orientations and placement within the existing experience, such as providing a separate tab on a job with recommendations listed, as well as automatically adding past candidates to the 'prospective' list with new, active candidates.
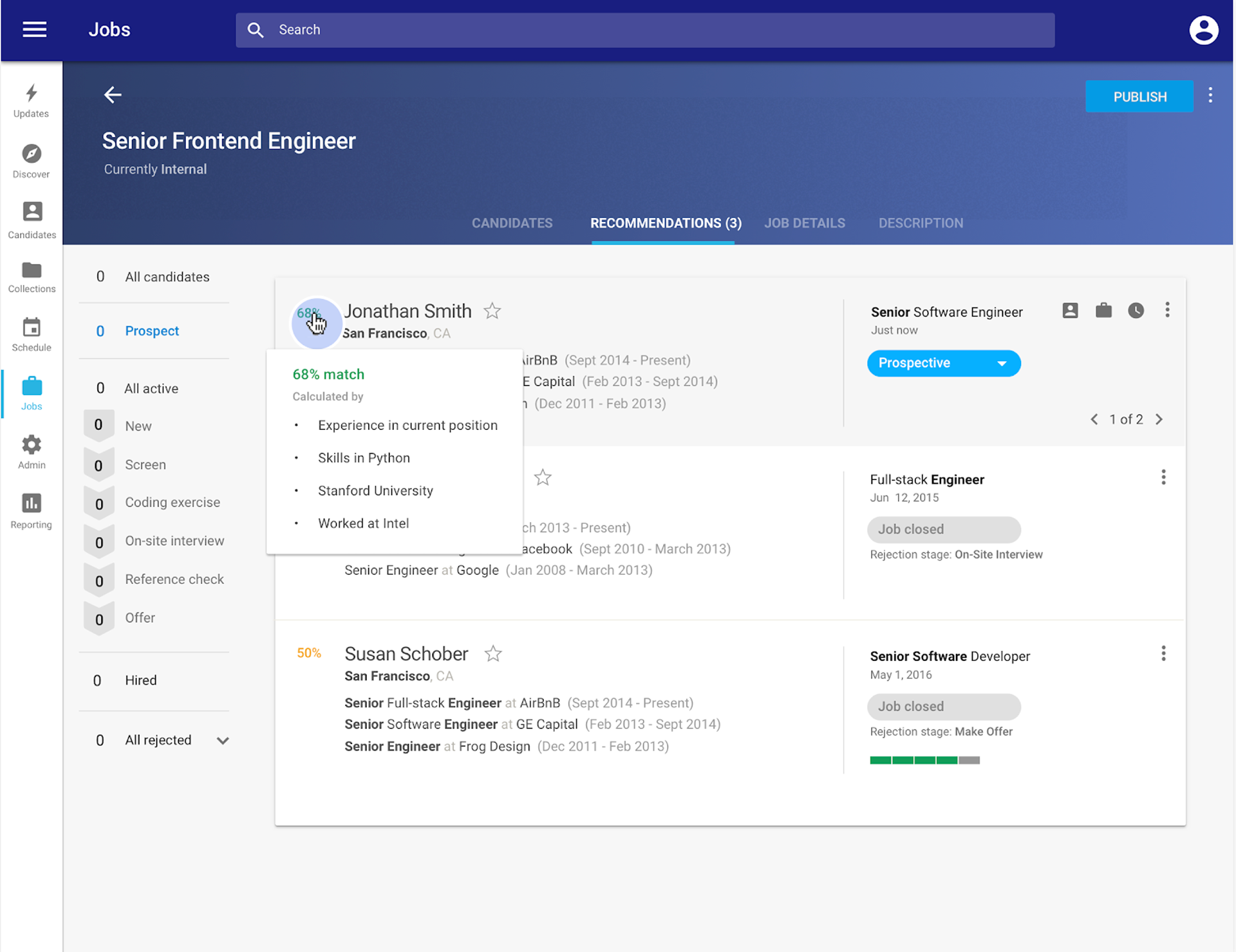
It was clear very quickly that recruiters wanted past candidates from the ATS in a separate area from the new candidate funnel to keep their focus on interested and active applicants.
“I just don’t want to get them (past candidates) confused with ACTIVE people who have APPLIED to this job.” (Participant 6)
It was also clear that a ranking system would be very helpful, as long as they (the recruiter) can configure the attributes they want to see in order to ensure matches are accurate and aligned with their expectations
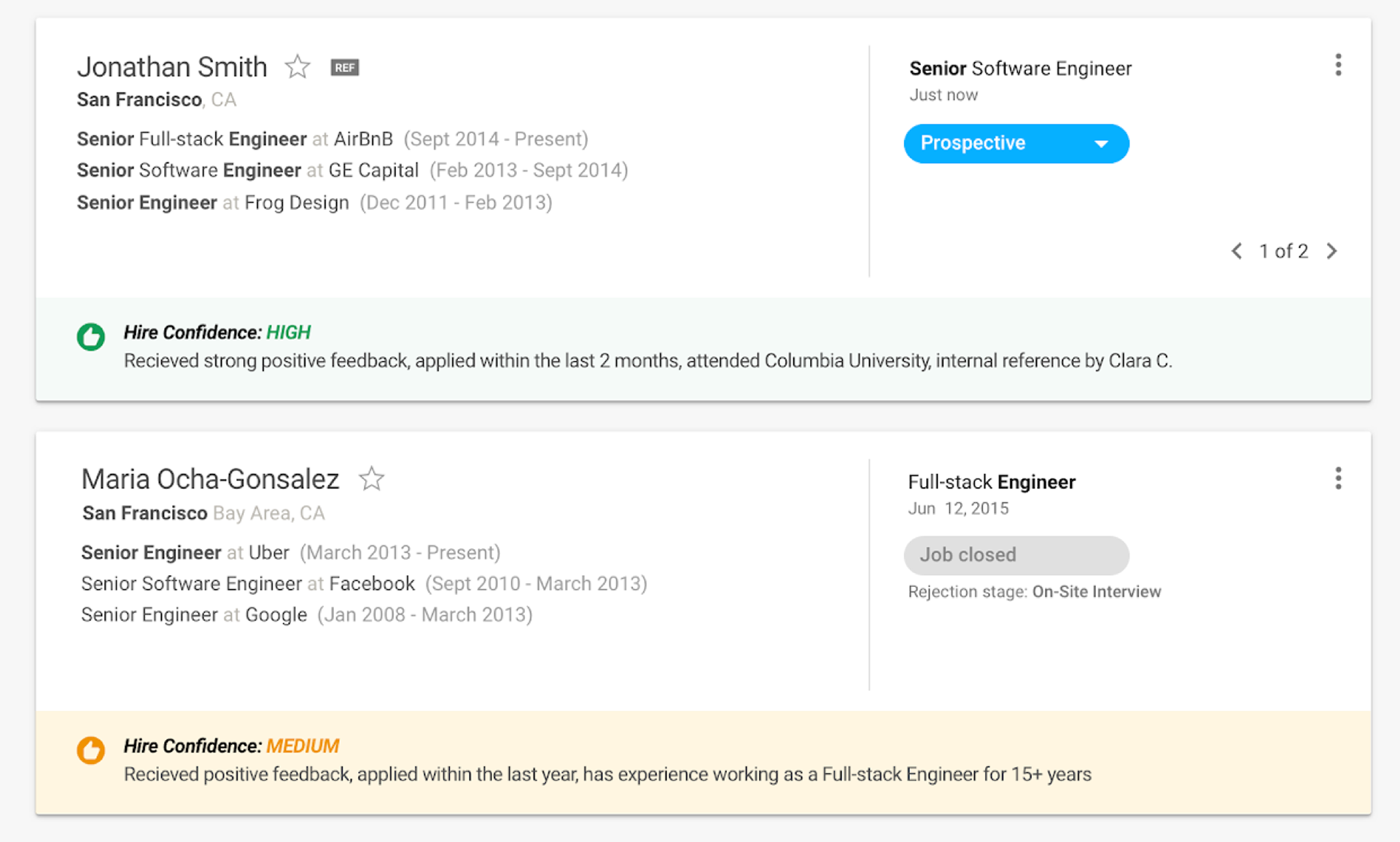
“There are plenty of people we would be excited to talk to from the past, but there are definitely people we would not want to talk to (1-2 ranking … i.e. low ranking). I would weigh previous interactions very highly.” (Participant 5)
Key findings
From our research, we gathered the following insights about the placement of the recommendations in the product:
•Recommendations are most helpful when they are in reach but don’t disrupt existing workflows
•Prefer recommended candidates not be accounted for in the “all candidates” total until added/specified manually
And in terms of a ranking or candidate match, we learned:
•Ultra-simplified representation of good/bad felt judgmental and didn’t provide enough context
•Recruiters want to see all candidates who are 90% or above in relevance first, but provide the option to see more via a collapse/expand button or a search results page in a different view
•Reduce cognitive load by showing less than 10 recommendations at a time, otherwise it’s overwhelming
•Ensure recommendations are not misconstrued as a reflection of the individual but of the quality of our ML to identify a match
Concepts
With clear findings from the research, I continued to develop ideas and iterate with the team on the best way to present the recommendations in the job view, on candidate profiles, and other areas of the product. It was also clear from the explorations, recruiters would also need to 'hide' candidates or remove recommendations from jobs where there was a 'bad' match. Having a more explicit way to inform and improve the matching algorithm over time would provide incentive to recruiters to provide feedback while also fielding candidates.
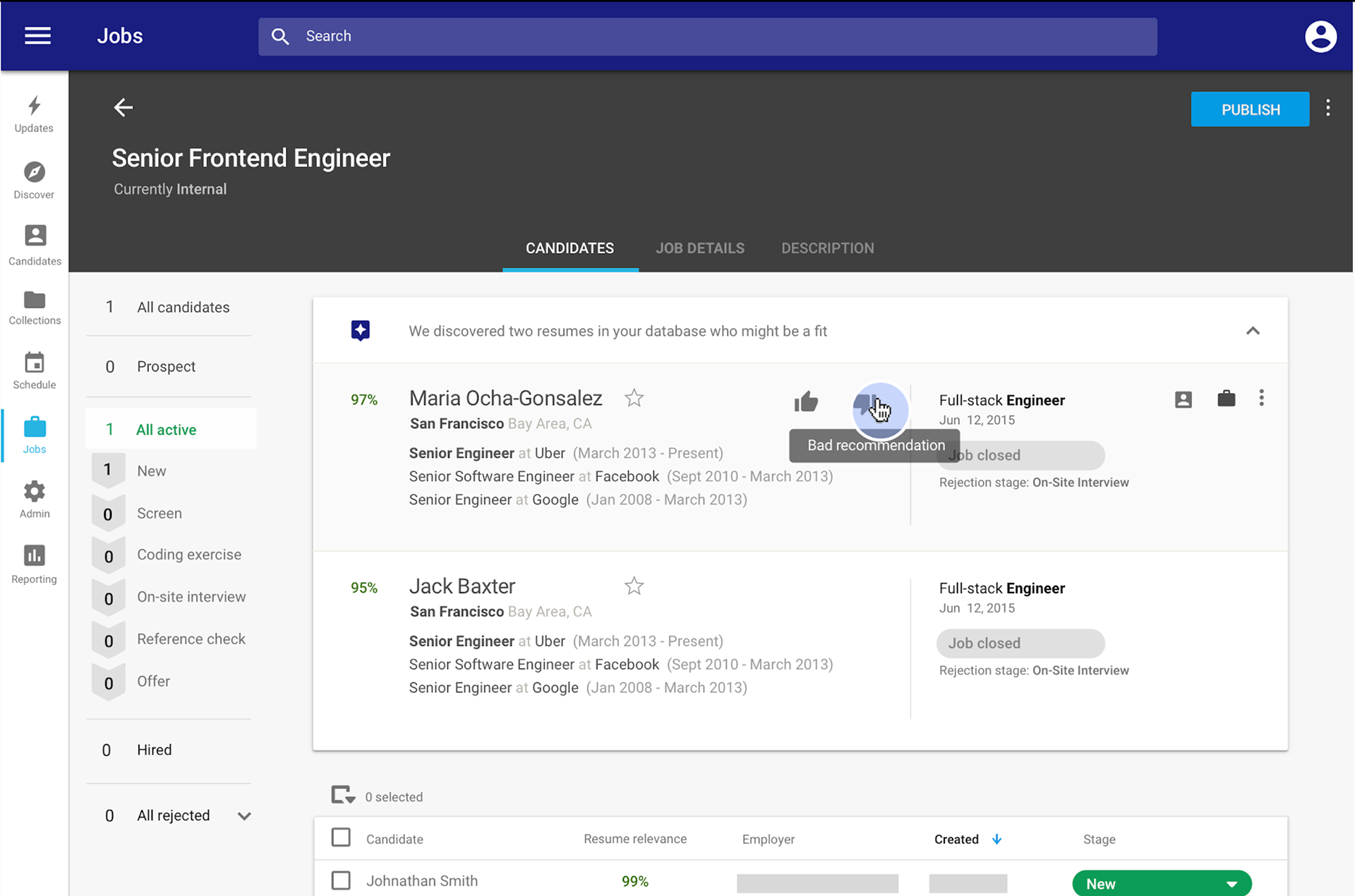
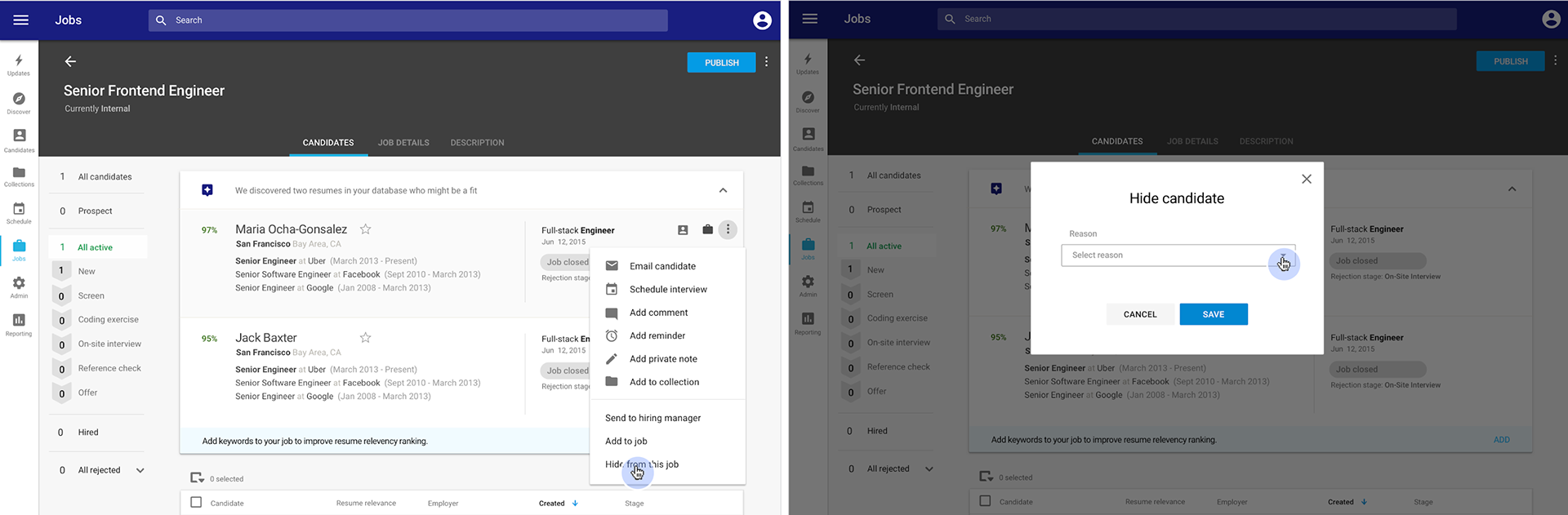
Shipped designs
My team released the beta 'resume match' feature to Google Hire's Trusted Tester network. The results of the experiment helped to determine next steps for the ML team and the viability of the 'match %' as a product differentiator for Hire. The rollout also informed overall quality improvements for candidate sourcing.
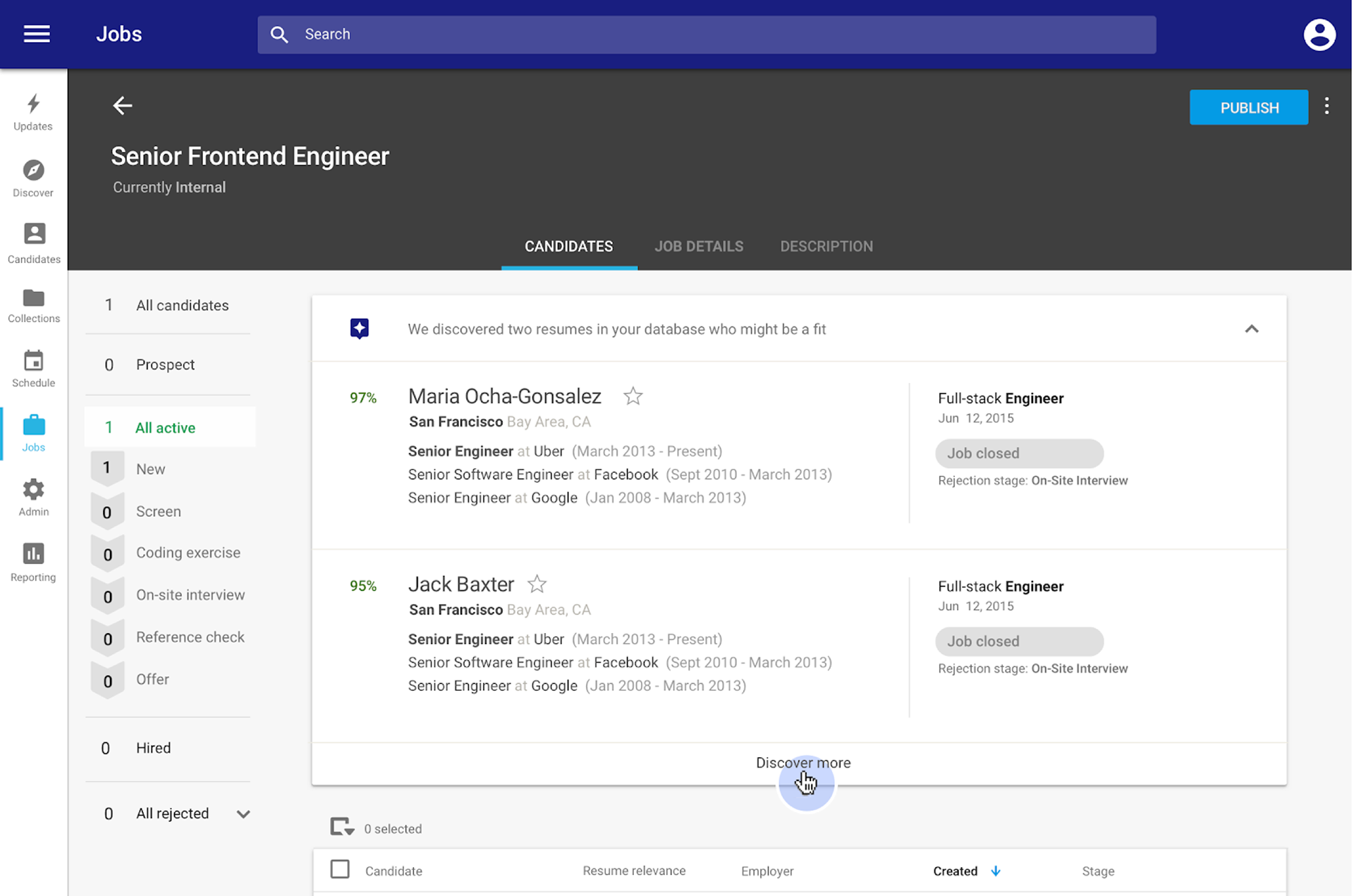
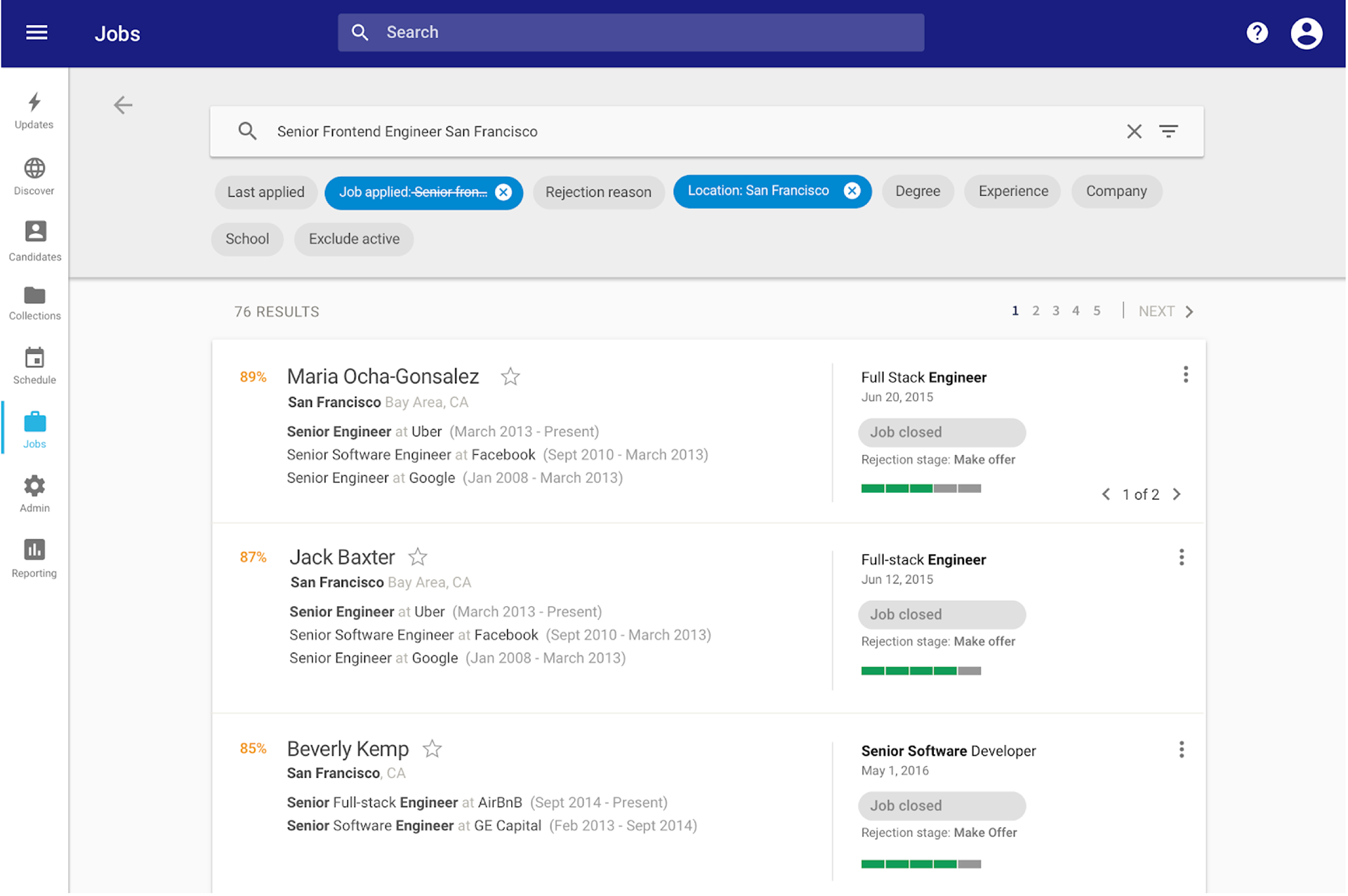
Project learnings and challenges
In developing any type of 'smart' automation tool for matching real people to jobs, there's a risk for bias that could cause harm or impact individuals unknowingly. As a designer, I tried to position match information in a clear and transparent way so it's evident how the system drew conclusions, with the ability to reject and configure attributes manually to override any defaults.